Exo Iris Lung and Cardiac AI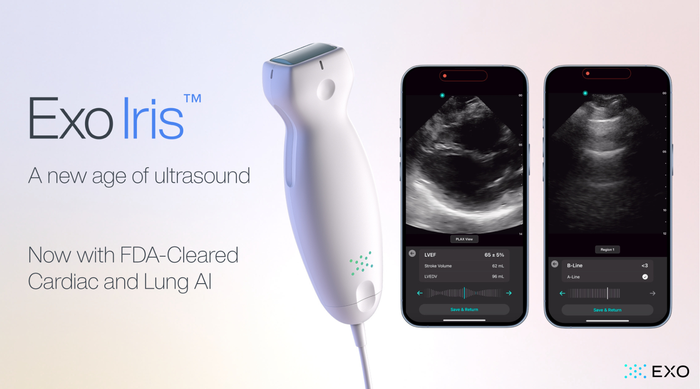
Artificial Intelligence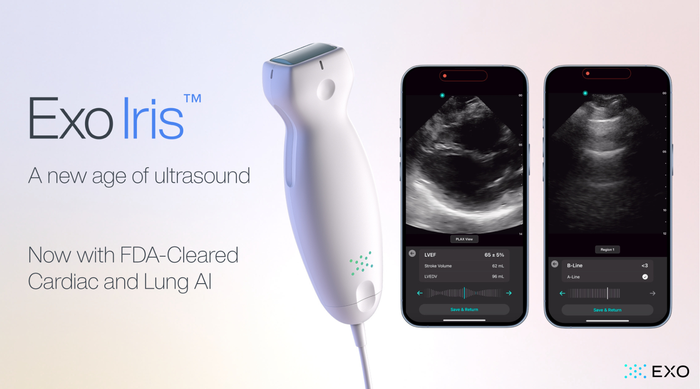
Exo’s FDA Cleared Cardiac & Lung AI Now on Iris Handheld UltrasoundExo’s FDA Cleared Cardiac & Lung AI Now on Iris Handheld Ultrasound
With the newly approved applications, Exo is now cleared for cardiac, lung, bladder, hip, and thyroid.
Sign up for the QMED & MD+DI Daily newsletter.