AI Doesn't Exist in Medtech, It's Just Machine Learning
Galen Robotics's CTO and co-founder, Dave Saunders joins Let's Talk Medtech to discuss why true artificial intelligence in healthcare doesn't exist...yet.
March 13, 2023
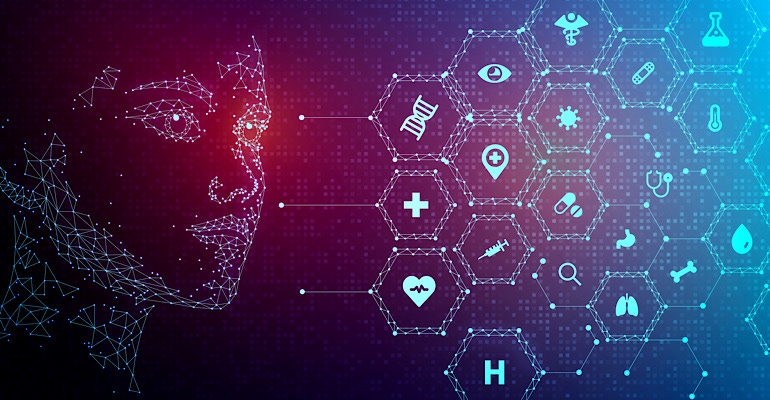
Don't call it aritificial intelligence - it's just machine learning. That's what Galen Robotics, co-founder and CTO, Dave Saunders is quick to point out whenever the initials AI come up. Saunders sat down MD+DI's Editor-in-Chief, Omar Ford on this episode of Let's Talk Medtech to discuss all things AI machine learning and discuss how the space was rapidly expanding in medtech. Oh, and we talk a little sci fi, too.
Episode Transcript:
Omar: Hello, and welcome to let's Talk Medtech, the premier podcast for the medical device and diagnostic industry. I'm your host, Omar Ford, editor in chief of MDDI, an online publication owned by Informa. On this episode of let's Talk Medtech, we've invited my good friend Dave Saunders, CTO and cofounder of Galen Robotics. He's going to be talking about the key difference between artificial intelligence and machine learning and why true AI. Artificial intelligence doesn't yet exist in med tech. This might be a controversial episode for some who would like to weigh in the other way on this debate, but at any rate, let's Talk Med Tech with Galen Robotics Dave Saunders. Dave welcome to Let's Talk Medtech Everybody knows that you're one of my most favorite people. I love talking to you. Every time we share a stage, we do a webinar. It's always a good time.
Dave: [Doing a] show or even running into each other on the crosswalk.
Omar: Yeah! In Boston, was it?
Dave: Boston, that's right!
Omar:Awesome. Well, listen, I want to talk a little bit about a word or two words that you hate, and I want you to explain that to our audience. But artificial intelligence, especially when it pertains to healthcare and medtech, why is that such a bad word for you? And you and I have gotten into this before. We've had this discussion on numerous platforms.
Dave: I don't know if hate is really the word, but typically when we're talking about AI or we're using that phrase, what we really mean is something called machine learning. And machine learning is just a small subset of the overall science of artificial intelligence, right? And so, machine learning is really just about taking in data and trying to process that data and making decisions based on it or offering decisions or offering different kinds of insight or analysis and that sort of thing. Now, the reason that the use of the word AI sometimes trips me up is because true AI needs to have motivation, right? It needs to actually have initiative and all those things that human beings have and machine learning doesn't. So, machine learning is just the tip of the iceberg for where AI is.
But as long as we understand that, when we're talking about AI, 90% of the time we're really just talking about machine learning. Like, gee, how does Netflix know what I wanted to watch next? And that sort of thing. We've got a pretty good context. And in healthcare, I think there are a lot of really amazing opportunities to apply machine learning or AI. And we've seen the effects of some of those already. And I'll just give you a couple of really quick examples that I think are amazing. So, one of those big imaging companies has a big CT scanner … sort of in the beginning of the COVID Crescendo, they got a software module approved for their CT scanner that was based on machine learning. And what it would do was you set it to CT scan of a patient's chest, and it would actually grade how much damage COVID had actually caused to their lung tissue, which is amazing. And now if you think about that in your lungs, you've got all the little bronchi and you've got just all this really intricate anatomy and you're trying to see it through a CT scan, which is designed to image bones, right?
So, it's a lot of weird murky shadows and sometimes some of the streaks are caused by builds up of mucus and things like that. Stuff that's not going to be very easy for a human being to process. And yet the effects of this machine learning module, from the research that I've read on it, have just been amazing, where it can just basically do triage for a patient. And you do a CT scan and it goes, this patient is in really, really bad shape. And compared to this person who appears that they can't breathe, well, this person over here is actually worse. And we can tell that because we're looking at their lungs and we're doing it with a system that can just see better than a human being can. And there are lots of opportunities. So, in genetic research, there's all this stuff about how DNA folds on itself and protein folding and drug interactions and all that kind of stuff. Machine learning is able to analyze that kind of information in a way that a human being would just be completely overwhelmed.
And there actually are online games for doing things like gene comparative analysis and protein folding. And some of those games were actually used to train the original machine learning modules that now do that stuff. And so, we know where a human being peters out and then we are able to see how machine learning is able to extend beyond that. Now, these are simple examples. I mean, they're not simple to do, but they're straightforward, very narrow applications of machine learning and where they're used, they're amazing. But here's the thing. No machine learning module is going to scream out, eureka, I just discovered a new drug and it's going to go and file the patents and come up with a new name and all that. There's no motivation behind it. It's going to produce information, it's going to produce a great analysis of data, but it still requires a human being combined with those results to really decide how to take action and in some cases, double check the work. Now, in my area, surgical robotics, I think we're much longer way away from seeing that happen. When you're talking about like DNA analysis and CT scans and things like that, your use is really narrow. But if you're thinking about that movie Prometheus, the Alien sequel or prequel, the woman got into the medical pod and there was no human being around in it.
Omar: Spoiler alert. We’ve got to say spoiler alert.
Dave Saunders: Right …spoiler alert … you've got an alien inside you, we're going to take it out, right? And it just did all by itself. Well, right now that is as science fiction as science fiction comes when it comes to the world of surgical robotics, we're not even close to considering that kind of autonomy in surgical robotics. So, there are plenty of areas in med tech where machine learning is just awesome, and then there are areas where we're not even close, and yet I sometimes read articles and I see comments on reddit and stuff like that where people are talking about these things as though we're a couple of years away. And I'm just like, shaking my head, going, no, we're barely at the point where you would trust a machine learning system to do a root canal. Actually, I don't think you would at all.
Omar: I want to touch on that for a second. Do you think we hit an inflection point? Not an inflection point, but do you think that there was a tipping point for machine learning? I want to say, rather than 2017 or 2018, where there was just such a hyper focus on it from medtech companies. And I've been covering this space for 15 years, and it seemed as if maybe in 2017, 2018, that's when things were really starting to kick into high gear with some of the larger strategics saying, hey, we're going to use, I'll say the bad words, artificial intelligence, machine learning. We're going to have this for our application. Do you think that the industry, even though there were probably strides that were happening before then, … do you think maybe in 2017, 2018, that was kind of around that time there was a tipping point, so to speak?
Dave: Yeah, there's a lot of motivation to do it right. There's a lot of motivation to see machine learning work. We've got eight billion people on the planet and counting, and I don't see hospitals growing in size at that same rate. So, it means we've got to find more efficient ways and cost effective ways of taking care of a wider population of patients. And that means that you really need to have more intelligent devices, help the human trained surgeons just do their jobs more consistently, more quickly and more cost effectively. There's a huge driver for that. Absolutely. And then when we look at the other side, I think some of the technology drivers google Cloud and Azure and AWS, those have been building up for quite a while now.
But I think they started getting to the point where we really were seeing demonstrations of, wait, I can just create an online account and I can just start running my initial versions of a machine learning system right here in the Cloud. I don't have to go and build a platform. I can just start to test it and what that created was an environment for all of these kind of Silicon Valley nerds that just go full tilt compared to medtech, and they're actually whipping out these software implementations and actually demonstrating their functionality. And a lot of companies got picked up during those years. There were a bunch of acquisitions. Companies are getting picked up. DeepMind was pulled into the Verb project for a little while with Google. And so, we saw a lot of that because there was a huge promise that the technology was finally there because, you know, machine learning obviously has been around for an awfully long time. I actually wrote a neural net in 1986, I think, based on an article from this old nerd magazine called Dr. Dobbs. I mean, so it's not like the algorithms haven't been around for a long time, but now we've got computing power. We've got, you know, bandwidth that is just ridiculous. You know, we're blasting, you know, 4K video, and we're complaining because we get a couple of dropped frames on our phone. And it's just like, it's amazing what we have at our fingertips and what we're capable of now consuming in terms of computing resources and what we're able to apply there. Now I think where we hit the wall was when those promises suddenly became logistically very difficult.
If you're doing a diagnosis, you're doing protein folding analysis, or you're an archaeologist, and you've got like a million and one pottery shards from a dig, and you just lay them out and you take a big picture and you say, machine learning, make me a vase. It's incredible what these things can do. It really is amazing. And that's one category. But when it comes to intervention, when it comes to, like, okay, I now want a robot that's going to look at this person, identify their appendix, and remove it all in one shot. Well, we're not there yet. And there are some amazing proofs of concepts that I've seen. For example, there's a project that was going on at Children's Hospital, I think it was just on a pig, where they did a reception of a colon, and then they had the robot actually do the anastomosis and rejoin the ends of the colon. And that was partially driven by a machine learning system, not just a pure traditional algorithm. Now, according to the research I've read, it took about 20 minutes longer just to do that suturing that a human being would have done. And yet all of the analyses of the end result were, this is probably the best suturing we've ever seen. I mean, it did a great job. It took a while to do it. Now, that's an amazing promise because it does tell us that at some point we're going to be able to solve some of these technology problems.
And then there's the other problems that I've talked about on stage before, but we're going to be able to get to that point where, yes, when the mission to Mars happens, there might be a medical bay that can do maybe some general kinds of surgery. Like, you get a big gash, you open up your bicep or something like that, and there's like 3 hours of tissue reconstruction. That might be something that's straightforward enough that you could teach a surgical robot how to do surgery for a glioblastoma. Maybe not, but there are things that it could potentially do and alleviate the responsibilities of who knows, the one surgeon on board. Right. And so, the potential is there. But yeah, it's funny that it happened just before COVID became an issue because honestly, I think from a market driving standpoint, COVID really brought home this notion of gosh. When you've got a situation where walking up to a patient could actually result in your own death, you really need to find some ways to provide.
I mean, we don't want to be completely isolating our patients. Human interaction is an important part of healing and just maintaining our constitution and things like that. But at the same time, if I can do things like drug monitoring and monitor all the vitals and then actually have a machine learning system that can put all of that data together and go, I think they're having interaction or I think this is happening. And either take action directly or alert a doctor or nurse or whatever to come and then take action. Those are things that we clearly need. And it's been said many times by the World Health Organization that this is not the last pandemic that we're going to see, and we need to be ready for those kinds of things. One of the biggest kinds of behind the curtain effects of the pandemic was there were things like aneurysms became elective surgeries.
Omar: Oh, yeah, those kinds of things.
Dave: Yeah, it was crazy. Now, if you look mechanically, I am no neurosurgeon, and I'm not going to pretend that I am one, but if you open a textbook and you look at like, okay, well, what do you do when you have an aneurysm? It's pretty straightforward. I mean, the actual skill required for a human being to work around your brain is off the charts. But if you look at it functionally, you go, okay, you basically have a bendy straw with a bulge in it, and I need to fix that. Right. There are a series of steps for repairing an aneurysm. Even though anatomy is very unstandardized, the actual steps that you need to conduct to correct that problem surgically are relatively standardized. So, you know what? If you could do some kind of triage and you could say, okay, this is a run of the mill aneurysm, for lack of a better phrase, the robot can handle this one because it's enough of a cookie cutter that the robot is not going to have any issues here. And then, oh, this patient over here. No, this guy's got a real issue. We need to make sure that a human being is there, the entire procedure hands on, right? And you start to do this kind of triage where you do start to have certain kinds of standardized surgical procedures where a robot might be able to handle things. Which means that now, not only is the human resources aspect of how many surgeons do you have at the hospital vs. how many patients on a particular day, that issue is alleviated to some extent. But then also you end up with this effect where yeah, we now can compartmentalize infection risk, we can compartmentalize exposure issues, and potentially, if there aren't human beings involved, it might even be possible. You can turn over the O.R. more quickly too.
Right, so there's a lot of benefits that are not super easy to quantify, but you start ticking them off on your finger and you're like, wow, this must be worth looking into. And sure enough, we look at all the medtech companies and then the myriad startups and folks out there that are looking at these problems and trying to come up with solutions for them. Yes, clearly there's a recognition that there's a big pot of gold at the end of the rainbow, which is in the form of a lot of patients getting excellent treatment in a timely manner. It's not that they're not mutually exclusive, right? If you help a lot of patients and you do good things, then obviously a lot of money is going to happen at the same time, regardless of what country you're in, somebody's making money when these things happen and so there is a great opportunity here and so there is a clear driver for it. It's a market demand, it's a technology push.
Everything has come together in such a way that we can reasonably, at least envision that we are within sight of actually building these technology solutions. Now. I think sometimes there have been initiatives that have been too aggressive, and they didn't even bother with the moonshot, they were just like, yeah, let's just go straight to Europa. And I think that's a little nuts. I mean, I always love to have big vision and stuff like that, but set your initial milestones to be a little bit more reasonable and actually prove that it can work first. But I think we're seeing that, and I think the opportunity is here more than it ever has been even ten years ago. I don't think we'd be having the same conversation. I think we'd still be talking about like handwriting recognition and eBay recommendations and stuff like that. That's really where machine learning was at and now, we're just seeing it way, way beyond that.
Omar: Well, Dave, let me break in and ask this. And that brings up a point that we've talked about this in the past, too, but when you look at the traditional, I don't want to say traditional when you look at the large strategics I don't want to drop any names because I don't want to get anybody in any type of trouble or anything. But when you look at some of the existing medtech companies and you look at the promise of machine learning and you look at the disciplines that these companies have been in in the past, how do you bring that new discipline of machine learning in? And do you change the atmosphere or the culture of the engineer of the company when you bring this new discipline in different this is new territory in a way.
Dave: Well, there are a lot of clash of culture issues when it comes to machine learning in med tech. First off, most of your nerds are over in Silicon Valley whipping out apps and auto caption things for TikTok and stuff, which is really cool, by the way. But they're knocking out apps where you can basically launch with a prototype and nobody's going to say boo. And then maybe you get a few angry tweets and you go, oh, that bug was a little worse than I thought, and you rev it up in the field and it's fine. Well, I can't do that with an EEG, right? I can't go through the regulatory process and then just, you know, the FDA says, well, what if there's a bug? And I'll go, I'll just push a rev overnight. It's not that simple. And so, you take highly, highly and it's not that these people are highly talented. It's just that the Silicon Valley culture is different, right? There's a bit more of a how do we say this nicely?
There's a bit more of a hustle mentality. And I don't mean that in a dishonest way, but you can move really fast, and you can make up for mistakes because you can push a new version to somebody's phone almost overnight, and half of your users wouldn't even have noticed that the update happened. And just magically things starts working and everybody's just like, hey, right? But in medtech, we have to be far more deliberate. There's a regulatory process, and right now when it comes to software running on a medical device, I need to be able to verify my algorithms. Algorithms effectively are mathematical equations. You can actually create a proof for an algorithm just like you do for a mathematical equation, right? And that's really important because I need to be able to prove to you that I can identify all of the risks in my software that runs my whatever. And I can tell you how it knows the difference between somebody's big toe in their appendix because you don't want to cut off the wrong thing that's sticking out. People get upset over that kind of thing.
Omar: They do.
Dave Saunders: Figure. So, you have to be very methodical in this now, bring machine learning into the mix. Okay, let's think about some of the early applications for machine learning and med tech a scanning interpreter for a Mammogram. And based on that scan, the machine learning algorithm can very quickly go, this woman is totally fine, completely clear. Send her home. No big deal, right? And this one over on this other end of the bell curve, holy cow, don't let this person even into the parking lot. Somebody needs to double check the scan immediately, right? So, there's the other end of triage, and then somewhere in the middle, there are other kinds of sorting. And then basically, you sort all of those scans, and then some human being, obviously, at some point, depending on what the grading is, will go, okay, I'm going to look at this pile tonight. That pile there are all the people that are supposed to be completely and totally clear. I'll do those on Friday because I've got time to do that. And those can apparently wait. And then, holy cow, here's this one that got flagged. Let's take care of this person immediately, right? These are diagnoses, but they still tend to get double checked by a human being as part of the overall workflow. And that's a reasonable thing to do. You look at, like, surgical intervention.
Okay, now what am I trying to say here? Let me see. You want to put a weapon in the hand of a robot, and based on machine learning, which doesn't actually have an algorithm that I can print out, you're going to tell me that it knows the difference between things sticking out of the body and it's going to cut off the right one. Obviously, we're referring to an appendix, and it's not going to make a mistake. It's not going to misidentify something else that might look like an appendix and cut it off instead. And the method that it uses to recognize what that thing is through the imaging system uses this thing called machine learning. And you cannot tell me, you cannot print out and create a mathematical proof like a regular algorithm that shows me with a high level of assurance that it always knows what the right thing is to cut off. And that's a weird situation for the FDA because they're like, so we're just supposed to trust it.
Now, keep in mind, for those who may not know that are listening, the way machine learning works is I feed it positive and negative data. So, if you want to develop a machine learning algorithm that knows how to identify pictures of cats, you give it a bunch of cats and you say, Those are cats. And then you give it a bunch of pictures that are not cats, and you say, Those are not cats. And then you start to let it respond back to you, and it goes, Is this a cat? And you go, yeah, that's a cat. Is this a cat? No, that's not a cat. It goes, okay, but is this a cat? You go, yes, well, after enough iterations of this and you can see proof of this, go to Google Images and type cat and some of those pictures that are clearly cats, you're like, Wait, a computer knew that that was a cat? Because some of them aren't that obvious. Like the cats are looking away or they're partially obscured, they're wearing a hat, I mean, all kinds of weird stuff. And yet the algorithm has just figured out what cats are, and you almost never see a false positive for image recognition. Well, that's great, but nobody's life is on the line. Nobody's appendage is on the line when it comes to having that recognized correctly, because even if it's correct, 999,999 times out of a million, nobody wants to be patient number one, where you have to come into the or the next day or come into the recovery room and go, so the robot cut off the wrong thing. Yeah, exactly, right. Medical errors do happen, but you can't sue a robot. And there's also a trust issue, right? People still feel that they can trust a human being more than they can a robot.
Even if the math says that the robot is right more than the human being, statistically, nobody wants to be the one. You always assume that. Yeah, but a human being would have figured that one out, right? And so, it's a really weird situation. So, there's a regulatory problem here, right? The current regulatory environment is not set up in such a way where I can bring a high-risk machine learning module into the or like this. I can use it to monitor vitals. I can use it to double check drug interactions, check out brain scans, all that kind of stuff. But right now, there is no regulatory pathway that's going to allow me to put the weapon, a scalpel, right into the hand of a robot and say, go cut this person on purpose. Right? It's not there. And so, there's a lot of regulatory work that needs to be done. I'm part of one lobby group that has been at least taking part in some of the work the FDA has been doing up to this point. There is, what do they call it, kind of like a preview document that says, this is probably how things are going to go. I forgot the word at the moment. It just happens. I'm getting old, man.
Omar: No, I hear you. Does FDA seem to be warming up to the idea of machine learning, in your opinion? They should be how to support it?
Dave: No, I think the FDA absolutely sees this as an inevitable market move into Medtech. I also think that it is clear to the people in the FDA, I think it's clear to them what the potential benefits of machine learning are, even to surgical robots. I think that the staff that is currently working on it is also a little overwhelmed because I've even heard things like, well, in order to actually fit machine learning into the current regulatory framework, we might need to rewrite the software regulatory framework from scratch. So, I've heard some of those comments being made, so clearly there's a lot of work that needs to be done, but there's also a lot of motivation to solve these problems because I think that they see it and they know that at some point. I mean, gosh, what was it? A few years ago, there was that surgeon in Antarctica, and he needed an appendectomy, and he was the only surgeon in all of Antarctica. Right. And so, what happened? He took out his own appendix. Right.
What if it was brain surgery? Right. This should not be your backup plan. There's a clear need to have autonomous, or at least semi-autonomous surgical robotics available in the marketplace that can at least relieve some of the burden or improve the consistency of procedures. Let me make this clear. I am not an advocate of replacing human beings with technology. But the way I see it is if we give human beings better technology, then human beings can do what they do great, which is be intuitive, motivated people that care about their patient and go, I'm going to make sure this person I understand what they're going through. They've got three different conditions or whatever, and I'm going to make the decisions that make sure that this person gets great care. But meanwhile, you could open them up and just throwing an example out. They could be littered with little micro tumors or something like that, right? You open up their abdomen, there's like a thousand of them.
Who's going to be better at counting 1,000? Hard to see little tumors covered with mucus and blood and then keeping track of their proper removal. A human being or a computer? What if they were both working together and the computer robot was actually tracking those things, advising the surgeon, hey, there's a cluster over there. Why don't we attack those next? They're highly vasculated or whatever, blah, blah, blah. And you go in there and you take care of that. And the human being is still very much in charge. They're calling the shots, but the robot is doing what it's great at doing as well, which is tracking tons and tons of data and keeping score. Right. And when we think about today's surgeon and when we think about tomorrow's surgeon, digital natives. And those are becoming more and more of the people that are working day in, day out. Right. Digital natives have grown up almost since birth having a high score or having a score somewhere in their field of vision. Right. Because they grew up with computers. They grew up playing video games. My seven-year-old behind me is out of school today. She's on her iPad and she's playing robots or something like that. This is something that they're just used to. Yeah. So, if you've got a robot helping you keep score. I think today's surgeon and even tomorrow's surgeon, they're not going to resist that. They're going to see that as a benefit. They're going to see that as motivation.
And so, I think the melding of next generation technology and still what humans are best at is a great mix. And also, the more of this, like, cognition stuff, heavy data analysis, tracking things, maybe doing a profusion test or something like that, all those things can be done really well by a robot. So let that cognitive load be released from the human being. And now more of their focus goes to intuitive patient care, applying their training, doing those things that they are the best at, and you get the best of both worlds. My analogy is always chocolate and peanut butter by themselves, they're both awesome. You put them together and it is my favorite candy. And so, they're not mutually exclusive things, humans and technology. Humans and technology have been going together for tens of thousands of years, and so this is just another progression of that. I just think we have to do it the right way. I know there are people out there that talk about replacing humans with tech, and I think there are some scenarios where you're going to do it out of necessity, but it's not my goal. I think the more you meld humans and technology, the better results you get.
About the Author(s)
You May Also Like