Harnessing AI: Key Considerations for SaMD Manufacturers
The critical considerations for SaMD manufacturers on how to take full advantage of the improvement power of AI/ML.
November 15, 2021
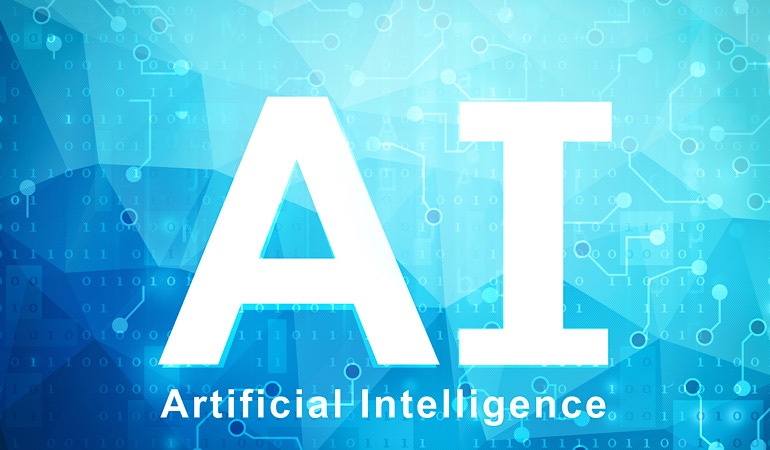
Introduction
A true artificial intelligence machine learning (AI/ML) software algorithm inherently improves as it goes, learning from every piece of data that comes in. So, imagine a world in which an AI/ML medical technology learns in the field, crunching new data points as quickly as it receives them to refine its own capabilities continuously and accurately in real time. The technology exists, and the roadmap is in the works among regulators and innovators. But this promising capability adds a lot of complexity for innovators and regulators to resolve before it can be unleashed and deployed in a health care setting to help clinicians make even better decisions.
To get there, medical technology companies must learn to harness this improvement power and prepare to take full advantage of this amazing AI technology. Here are three important ways to get started.
1. Be specific on the value proposition
Currently, the algorithms fueling in-use medical products are “locked,” meaning the version of the algorithm doesn’t change in the field, even as new data points are received and, in most cases, uploaded to the cloud to inform the product’s next generation. The challenge for the U.S. Food and Drug Administration (FDA) is to balance the assurance of safety and effectiveness, while getting the benefits of this continuous self-improvement learning capability. However, the FDA’s traditional way of reviewing products isn’t formed for this, and, so far, the FDA doesn’t have a standard way to regulate an unlocked algorithm in field use. Before allowing self-modifying and self-improving AI medical technologies to be deployed, regulators will need to understand how the underlying algorithms are appropriately and reliably verified and validated.
To this end, innovators need to make it as easy and direct as possible for the regulators to assess the safety and efficacy of a given AI medical product. The tendency among product developers is to think the software will tell you what you need to know. But for the purpose of an FDA-regulated AI medical product, it won’t, and you don’t want it too.
Rather, you want – and need – to anticipate exactly how it will work, how it could change, and what the results will or might be. This means starting with a very specific value proposition and being crystal clear about what the algorithm is going to do, and how that translates into a claim or clinical end point. You must be certain and specific, because after the fact, you will need to be able to monitor and manage the performance of that algorithm for any type of change that comes from the inherent iterative power of machine learning.
2. Embrace the Agency partnership
Initially, medical device manufacturers were concerned the FDA would handle each new iteration as a discrete event and require an approval resubmission. However, in the interest of providing more and more value to patients, the FDA has steadily provided “steps and landings” for innovative company’s interested in developing AI/ML medical products. Just in the past few years, for example, the FDA has:
Issued a discussion paper with a request for feedback on a proposed regulatory framework for modifications to AI/ML software as a medical device (SaMD) (April 2019);
Proposed and published a Digital Health Innovation Action Plan (March 2020);
Implemented the Digital Health Software Precertification (Pre-Cert) Program (updated September 2020);
Published an Artificial Intelligence/Machine Learning (AI/ML)-Based Software as a Medical Device (SaMD) Action Plan (January 2021); and
Launched the Digital Health Center of Excellence to empower innovators and make all related information easier to find.
Furthermore, as part of the Action Plan released in January 2021, FDA introduced a proposed framework that allows for iterative improvements, building on concepts from the Pre-Cert Pilot Program for SaMD and expanded on the concept of a predetermined change control plan. Importantly, the predetermined change control plan envisions that the manufacturer has control over and anticipates how the AI/ML-based SaMD will change. The plan allows the manufacturer to execute the change via change control processes in their quality management system without having to go to FDA through a new 510(k) submission. For this, FDA is taking a stepwise approach. The framework currently only applies to 510(k) products (Class II), and not for pre-market approval (PMA) products (Class III).
This conceivably sets the stage for future innovations. Once more experience is gained, the FDA could open the framework to include PMA products and allow for predetermined changes under a plan to be included in an annual report, for example. From there, with even more experience, FDA could eventually open the use up for “unlocked” algorithms, such as first through scenarios whereby the ML algorithm is unlocked for changes within set guardrails identified in a predetermined change control plan.
And that is the thing – as painstakingly detailed, complex and at times frustrating as going through regulatory approval processes can be for all involved, when it comes to AI/ML, the FDA has and continues to be a strong, proactive partner of the industry. Essentially, the Agency is doing what it can responsibly to enable innovative companies to develop adaptive and evolving AI/ML technologies.
3. Change the industry
The Agency has provided these landing spots, but it is up to innovators to stick the proverbial landing by considering the product’s dynamic lifecycle from inception through obsolescence. That requires determining how to control data inputs, including new ones being amassed in the field, what and how the machine will continue to learn and change, and how to evaluate and verify the results of that learning to maintain the product’s integrity and defined purpose. And that requires changing the traditional individual mindsets of medical technology and software development companies.
Most software requires far less pre-market documentation, testing, and validation than is mandated for regulated AI/ML medical technologies. That being the case, software companies usually develop products in calculated sprints with an aim to get a decent software to market as fast as possible. From there, just as it is developed in sprints, software tends to be revised and updated in sprints post-market. This agile process doesn’t work as well for regulated AI/ML medical products in which safety and efficacy are – and should be – prioritized above all else. That said, FDA has worked with industry and standards development organizations to address how agile development might be altered to address FDA quality system requirements. [For an example, see AAMI TIR45:2012 (R2018) – Guidance on the use of AGILE practices in the development of medical device software.] So, while some software giants already are making these adjustments and investing heartily in building out their internal teams and infrastructures to meet the due diligence needs of regulatory compliance, many others will be forced to play catch up.
Conversely, medical device companies are accustomed to the detailed rigor of the regulatory approval processes, but they generally lack the ingrained agility of their software development counterparts. So, to compete in this AI/ML space of the future, medical device companies will need to figure out how to develop products faster without jeopardizing compliance.
In either case, change is necessary. Both need to rethink how they approach research, development, and commercialization of AI/ML software in order to be relevant.
Moving forward with clarity and purpose
Fulfilling the promise of AI for SaMD presents unique challenges. Clearly, the Agency has shown it is and remains committed to encouraging innovation. So too must innovators. Innovators need to take responsibility and hold themselves accountable for innovating with evidence-based purpose and reliably provable outcomes. They must do their part to embrace the Agency partnership, participate in the industry conversation, and look for reliable ways to leverage the continuous learning capability of AI/ML technology.
No one knows exactly what the future holds, but, as innovators, we are all in this together. The farther we can push technological advances to safely and efficaciously realize the huge promise of this amazing technology, the more people will be empowered and enabled to live healthier lives.
About the Author(s)
You May Also Like