The Challenges That Big Data Has to Overcome
Big Data holds a lot of promise but first it has to overcome some major challenges.
June 2, 2016
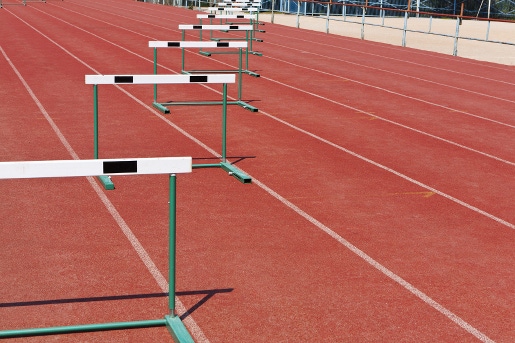
Arundhati Parmar
Payers, hospitals, doctors, healthcare companies and innovators alike are deeply excited by the promise that Big Data holds in transforming medicine through predictive analytics and precision medicine.
But there are some shackles that are hampering Big Data from realizing its true potential, believes Peter Winkelstein, executive director, Institute for Healthcare Informatics at the University of Buffalo.
"How you take raw data and turn it into something clinically useful for a doctor or to a clinician or patient themselves is a hard problem," said Winkelstein, who will be talking about Big Data and connected devices at the MD&M East Conference, June 14-16 in New York.
Stay on top of medtech trends and attend the MD&M East conference at the Jacob Javits Convention Center in New York, June 14-16. |
While individual efforts in certain kinds of Big Data are being made -- Medtronic working with IBM Watson Health to create an app using data from insulin pumps and continuous glucose monitors that can help diabetes patients manage their disease better -- the entire field will benefit if these challenges could be overcome.
Here are some top challenges:
Lack of Interoperability
The lack of technical standards for how electronic medical records talk to each other is a big hurdle, a longstanding problem.
"Hospitals' electronic health records may be different from the urgent care's health record which is different from your own doctors' health records and they don't talk well," Winkelstein explained. "They don't move data around well."
The government - agencies like Office of National Coordinator and FDA - has stayed away from imposing standards on industry because it appears that it is looking at professional societies to come up with standards, he said.
There are some standards out there around some of the data ... but it's a very complicated problem and I would like to see the informatics community take the lead in this," he said. "There have been some attempts ... but what I am beginning to see is that electronic health record companies essentially are trying to do it themselves because they've got to do it."
Difference in How Data is Stored
Imagine that the interoperability and data access problems are solved - big stumbling blocks that they are - there is another hurdle for Big Data.
"Even if you can get the data from the electronic health record, you then have the problem that the data is stored potentially differently in each of the different electronic health records and you have to somehow match up data elements that ought to be in the same place," he said.
Take for example blood pressure that might be entered differently in each of the electronic health records housed within those different healthcare facilities.
"Blood pressure is typically two numbers systolic and diastolic, so say 120 over 70, so how do you store that? Do you store a systolic blood pressure different from a diastolic blood pressure? Do you store it as a single text field with a systolic/diastolic number which would be the way if somebody wrote it down? Is any normalization done because blood pressure varies if you measure it standing up, sitting down, lying down? Have you recorded the position of the patient - does anybody care about it?"
Winkelstein says that these questions become more complicated when it comes to labs, and then even more so when doctors enter clinical notes following a patient visit.
Lack of Strong Statistical Models
Winkelstein believes that the some of the statistical, data processing techniques out there that are not appropriate to analyzing large data sets that are the very heart of Big Data.
"Correlations are a good example that don't lend themselves well," he said. "P values are all about looking at whether or not two values are correlated by chance. Well, when you have an enormous data sets, you are going to find some values that are correlated and they don't mean anything. It's just chance but we don't yet have the statistical techniques to sort through that very well."
So how can the power of Big Data be unleashed and the above problem solved. Winkelstein pointed to another tern: ontology. In information science, ontology is an ontology is a formal naming and definition of the types, properties, and interrelationships of the entities that really or fundamentally exist for a particular domain of discourse, according to Wikipedia.
In healthcare, we need to create medical ontologies.
"Ontologies are ways of encoding the data so that you can understand the relationship between the data points," Winkelstein said. "So we need much better medical ontologies and that will help to solve the standards and the interoperability problem. If I could wave my magic wand, I would say let's produce a really good medical ontology because that would in many ways free the data from its current constraints and a lot of noises in the current data.
Arundhati Parmar is senior editor at MD+DI. Reach her at [email protected] and on Twitter @aparmarbb
[Photo Credit: iStockphoto.com user maximult]
You May Also Like