Next-Gen Test Uses Robotics & AI to Predict Responses to Cancer Drugs
The PARIS Test prescreens a broad panel of cancer drugs in patient-derived live tumor cells and provides actionable results 70% of the time, on average.
October 8, 2021
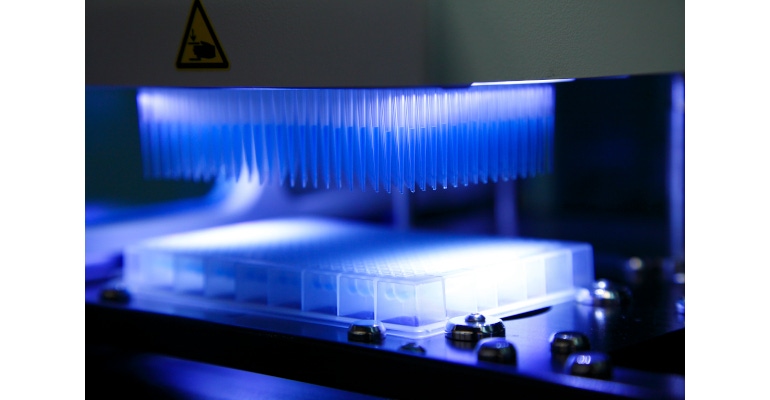
Almost always, cancers have multiple coexisting mutations, which makes finding a drug to treat them very difficult. “We're so used to thinking. ‘Oh, HER2 breast cancer, Herceptin,’ but that's the rarity,” said Carla Grandori, MD, PhD, co-founder and CEO of SEngine Precision Medicine, in an interview with MD+DI. “Most of the time there are multiple drivers, multiple weaknesses.”
SEngine has developed a novel way to find a targeted treatment for individuals with these complex cancers using robotics and machine learning. The first step of the company’s PARIS Test is taking a biopsy of the cancer cells. These cells are kept alive in 3D and at the correct physiological oxygen concentration, basically growing mini-cancers in a dish. “That's what is different [from previous technologies],” said Grandori. “We have experimented and defined specific conditions to keep cancer alive ex vivo from greater than 30 tumor types.”
The next step is putting the tissue in microtiter wells. The PARIS Test is done robotically in the laboratory, because, as Grandori put it, “as humans we make too many mistakes and it would take a hundred-fold-more time!
“Once we do the assay, we expose [the sample] to a reagent that emits luminescence that can be measured and that tells us how many cells are surviving or not after the addition of drugs,” she said, noting that multiple drug concentrations are used. “We have been very, very careful to assemble a library that is the most up-to-date drug library with hundreds of oncology drugs,” she said. The library presently totals 250 drugs.
The PARIS Test is 70% actionable. “Actionable means that 70% of the time, even [with] patients who have exhausted their standard of care, we can find a drug match,” she said, explaining that in comparison, the actionability of DNA sequencing ranges from a low of five to seven percent to a maximum of 20%.
“I think where we are most unique is that we have revolutionized how we measure drug response,” Grandori said. She said that machine learning and other metrics are included in how the PARIS Test judges and ranks drug responses for each patient’s cancer cells ex vivo.
“That is a major advancement that has led us to come up with a score and a series of categories that is part of the report that goes to the oncologist,” she said. “We're excited to help patients and oncologists identify potential treatments, especially in situations where the standard of care fails, or to make decisions among the different standard-of-care drugs.”
One recent patient story Grandori tells is of a woman with terminal ovarian cancer who was tested with the PARIS Test and matched with a drug previously only thought to be useful for lymphoma. The patient’s cancer marker CA125 decreased by half within one month after treatment, and in six months she was well enough to travel on vacation.
“That story tells us that there are these targeted therapies that can be very effective with much less toxicity,” Grandori said. “Even in a case where that tumor is so resistant to the broad chemotherapies.”
Grandori concluded by saying that besides finding drug matches for patients, she thinks the PARIS Test platform could be used both to prescreen experimental drugs to gain information on cancer indication and biomarkers predictive of drug response, and to accompany clinical trials, increasing their success. “We have executed already some of these collaborations [with pharma and drug developers] where they might have a preclinical candidate and are tested across 200 patient-derived organoids,” she said.
Grandori also believes that, in the future, the technology could be broadened to work with any condition for which there is a cell model, such as cardiovascular, neurological, or muscle degenerative, or hematological diseases.
About the Author(s)
You May Also Like