In Medicine, Small Steps — Not Moonshots — Will Advance Enterprise AI
Artificial intelligence needs to walk before it can run, especially in healthcare.
December 11, 2020
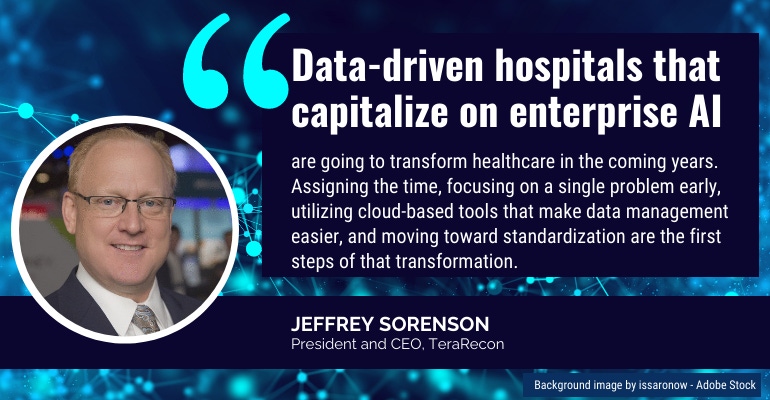
Jeff Sorenson, President and CEO at TeraRecon
Before AI runs it needs to learn how to walk, especially in sectors like healthcare where trust and reliability is often a life-or-death question.
Physicians and administrators have seen enough failed AI moonshots to be skeptical of products that promise to “transform” or “revolutionize” health care.
What all medical professionals want — and what the world’s leading medical centers are deploying — is targeted solutions for particular pain points; products tailor-made to support specific clinical use cases.
As clinicians become comfortable with AI, and convinced of its efficacy, we can start to realize its wider use and transformative possibilities.
No big bangs
Starting small reveals the capacity of enterprise AI to mine data in ways humans can’t. Administrators and physicians should start with one solution in one business or medical unit and focus on a single aspect of their workflow or practice. As this happens, health systems will require flexible software platforms that are purpose-built for the first use case and can expand to an increasing range of advanced AI-powered clinical tools. Today, medical imaging provides one the most fertile grounds for cultivating progress.
Progress is made outside of imaging as well. Mount Sinai Hospital, one of the country’s most renowned teaching hospitals with some 50,000 inpatients a year, is a pioneer in this start-small philosophy. Last year, it deployed powerful algorithms to detect nutrition deficits among its patients, increasing diagnoses by 20%. Another AI project sought to identify diabetic patients at near-term risk of kidney disease, who could then move to the front of the line for specialist visits.
AI will most certainly transform Mount Sinai and other flagship medical centers in the years ahead, but it won’t happen in one fell swoop.
Too many health systems try the “Big Bang” approach, attacking whole complex systems at once and flaming out fast under a mountain of novel observations that don’t relate to preexisting strategic goals. Starting small and moving incrementally, in contrast, make for lasting improvements.
Choose discrete projects with fast cycle times and potentially big or at least clear and noticeable impacts. That process might require internal surveys that delve deep into the institution. Or it might require executives to undertake honest conversations about challenging, intransigent or neglected parts of their strategic agenda. The point is, organizations need to make space to get this going.
AI in healthcare — where to start?
We encourage hospital leaders to revisit macro changes that have impacted their game plans. That’s where administrators and doctors are likely spending the most energy dealing with problems that they can identify but can’t quickly or easily solve. It seems like a simple formula, but combing through an organization and seeing where that dynamic friction is taking place can be challenging.
With the pressures of value-based care and reimbursement, readmissions were a logical place to focus for Northwell Health, New York state’s largest health care provider. Last year, the system integrated a readmission-focused “predictive artificial intelligence software” into its Electronic Medical Records at 15 hospitals.
The aim was to digest huge amounts of patient information with the purpose of identifying factors contributing to readmission, through scouring medical histories and accounting for social determinants, such as transportation or housing.
A similar project was undertaken at the Baltimore-based University of Maryland Medical Systems, using the “Baltimore score,” a number based on 382 variables that helps predict readmissions before they happen.
Those variables include demographics, lab test results, if the patient required breathing assistance, body mass index, affiliation with a specific church, marital status, employment, medication usage and substance abuse, according to Becker’s Hospital Review.
The “B score” more accurately predicted readmissions than other scores. "This study underscores how patient data may also help solve the readmission puzzle and, ultimately improve the quality of patient care,” E. Albert Reece, dean of the University of Maryland School of Medicine, was quoted as saying.
As hospitals dig down into this data focused on readmissions, they will likely find new insights into where they need improvement — possibly sparking their next AI adventure.
Data-driven hospitals that capitalize on enterprise AI are going to transform healthcare in the coming years. Assigning the time, focusing on a single problem early, utilizing cloud-based tools that make data management easier, and moving toward standardization are the first steps of that transformation. The insights to come will conserve resources and save lives.
Jeff Sorenson is president and CEO at TeraRecon. Prior to TeraRecon, he held leadership positions within the healthcare verticals of Hyland Software, GE, Imatron, and Philips.
You May Also Like